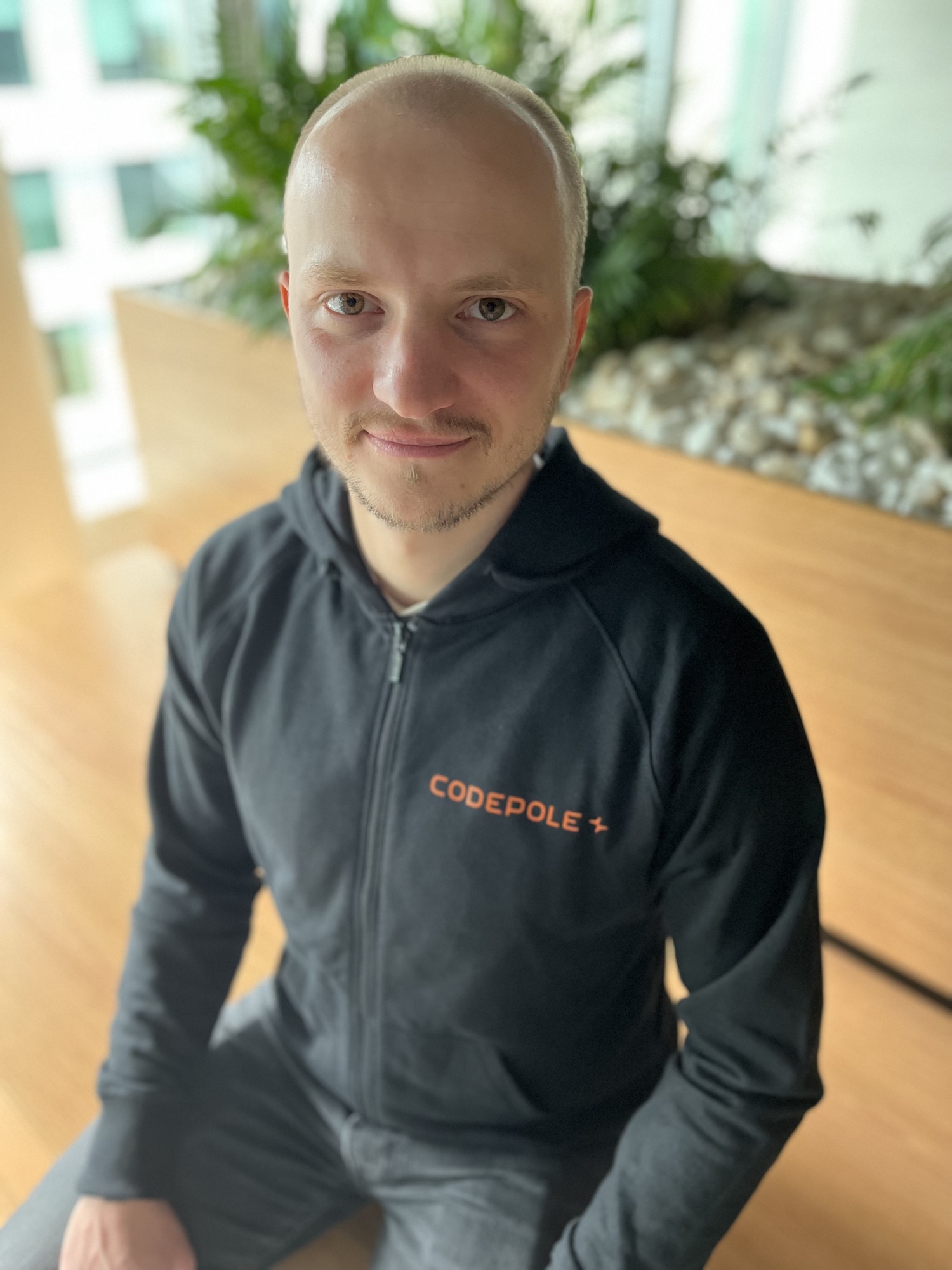
ParetoLabs is a startup named after the Pareto principle, emphasizing the efficiency of 20% of input yielding 80% of results. Currently, it is a small team led by Tobias and Ahmed, aiming to grow gradually alongside the technological developments in AI.
Our discussion delved into the evolution of technology trends, referencing the Gartner Hype Cycle and the current status of blockchain, NFTs, AI, and ChatGPT. ParetoLabs advises clients to experiment and adapt to technological advancements, emphasizing the importance of data quality and timely adoption.
ParetoLabs tech
The presentation by Tobias introduced Large Language Models (LLMs) and focused on ChatGPT's capabilities. ParetoLabs has developed an AI mid-layer to manage context, LLM, and interfaces, incorporating process integrations. The retrieval augmented generation method was detailed, and explained in more details including the different parts: a knowledge base, input and output questions, and relevant context retrieval.
The data into the vector database is populated through data scraping, exemplified with a railroad company use case. ParetoLabs suggests a balanced approach to token chunks for effective retrieval. The company emphasizes retrieval augmented generation as an efficient way to employ LLMs for various purposes without the high costs and limitations of frequent retraining.
ParetoLabs shared its experience with Rhätische Bahn, a Swiss train company, using retrieval augmented generation to simplify complex ticketing systems. The technology is also applicable in the financial sector for in-depth client due diligence. The first part of the presentation concluded with an exploration of diverse applications, from chatbots to text analysis, showcasing the versatility of retrieval augmented generation powered by large language models.
Customisation of ChatGPT
The discussion continued with a demonstration of how retrieval augmented generation works for OpenAI Premium users, showcasing the new custom GPT builder feature. Tobias compared their tool with OpenAI's custom GPT builder, highlighting its ease of use and efficiency in creating a retrieval augmented generation pipeline.
The custom GPT builder allows users to configure descriptions, instructions (prompts), inquiry handling, interaction styles, browser usage, and additional content. It also supports capabilities like web browsing, image generation, code interpretation, and linking actions through JSON format for API usage.
Tobias emphasized the power of custom GPTs, drawing parallels with their AI mid-layer, which coordinates large language models (LLMs), interfaces, and process integrations. He encouraged us to try out the tool ourselves :)
The live demonstration involved asking customised chat bot questions related to a train company and testing the system's responses. It showcased how the custom GPT efficiently handled queries but also highlighted potential pitfalls, such as the model's overconfidence in providing factually incorrect answers.
Factual correctnes and limitation of Large Language Models
The discussion further explored the challenges of ensuring factual correctness and alignment, especially when dealing with sensitive information. Tobias demonstrated the need for careful prompt engineering and continuous testing to fine-tune the system's performance.
When discussing controlling the scope of the AI's responses, Tobias acknowledged the challenge of defining clear boundaries in a gray area of responses. Further, we discussed the need for iterative prompt adjustments and emphasized the ongoing exploration of ways to monitor and test the system for accuracy.
The conversation then shifted to data privacy concerns, discussing the anonymization and protection of sensitive information when interacting with large language models. We touched on potential implications and uncertainties surrounding the use of personal data in the development and training of AI models.
The presentation concluded by touching on the evolving landscape of AI, with the integration of custom GPTs into an App Store-like ecosystem. Tobias highlighted Microsoft's approach, referring to copilots and the Power Virtual Agents platform. We shared curiosity about future developments and emphasized the increasing role of AI in diverse fields and the potential for individualized solutions.